Internal Publication
Land use/land cover classification for the iron ore mining site of Kishushe, Kenya: a feasibility study of traditional and machine learning algorithms
Motivated by the need to enhance the precision of land use/land cover classification for mining environments challenged by rapid anthropogenic and natural changes, we analysed multispectral Sentinel 2A satellite data using four different classifiers: Maximum Likelihood Classifier (MLC), Support Vector Machine (SVM), Random Tree (RT) and Random Forest (RF). Using adjusted training sample sizes drawn from the Kishushe iron ore mining site in Taita Taveta, Kenya, we conducted image analysis and compared the classification accuracies of the four methods, confirmed further by ground truthing. The study met the main objective of evaluating and comparing the performance of the traditional Maximum Likelihood classifier with the three machine learning algorithms of Support Vector Machine (SVM), Random Trees (RT), and Random Forest (RF). Eight land use/land cover classes were generated from each of the four classifications performed in R statistics software for RF and in ArcGIS 10.7 for RT, MLC and SVM methods. Random Forest (RF) method delivered the best overall accuracy at 74.63 % with a Kappa value of 0.67. Random Trees (RT) method came second at 72.64 % with a Kappa value of 0.64. The overall accuracy of the SVM method was 58.21 % with a Kappa value of 0.46 and for the MLC method, the overall accuracy was 57.21 % with a Kappa value of 0.45. These results confirmed that machine learning classifiers outperform traditional classifiers. The study also confirmed that for robust land use/land cover classification, it is essential to have quality training data as the quality can have large and considerable effects on classification results. Since the reliability of land use/land cover (LULC) maps derived from remotely sensed data for mining sites depends on accurate classification, this study gives evidence-based recommendation for adopting machine learning algorithms in satellite image analysis and classification to support environmentally sustainable decisions and informed policy direction for sound mine planning and monitoring.
Uploaded by: Nashon AderoAuthor: Siljander, M.
Co-author: Gitau, F.
Co-author: Nyambu, E.
Co-author: Adero, Nashon | ORCID: 0000-0003-2830-7912
Institution: Taita Taveta University College | Centre: Kenyan German Centre for Mining, Environmental Engineering and Resource Management (CEMEREM)
Type: Journal article | English | Peer Reviewed
Subjects: Mining
Published in: African Journal of Mining, Entrepreneurship and Natural Resource Management (AJMENRM), ISSN 2706-6002 | volume 1, issue 2
Publisher of document: Kenya : Centre of Excellence in Mining, Environmental Engineering and Resource Management , Taita Taveta University
Date: April 2020 | Pages: 115-124
Copyright: © 2020, AJMENRM | License: AJMENRM, All Rights Reserved
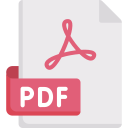